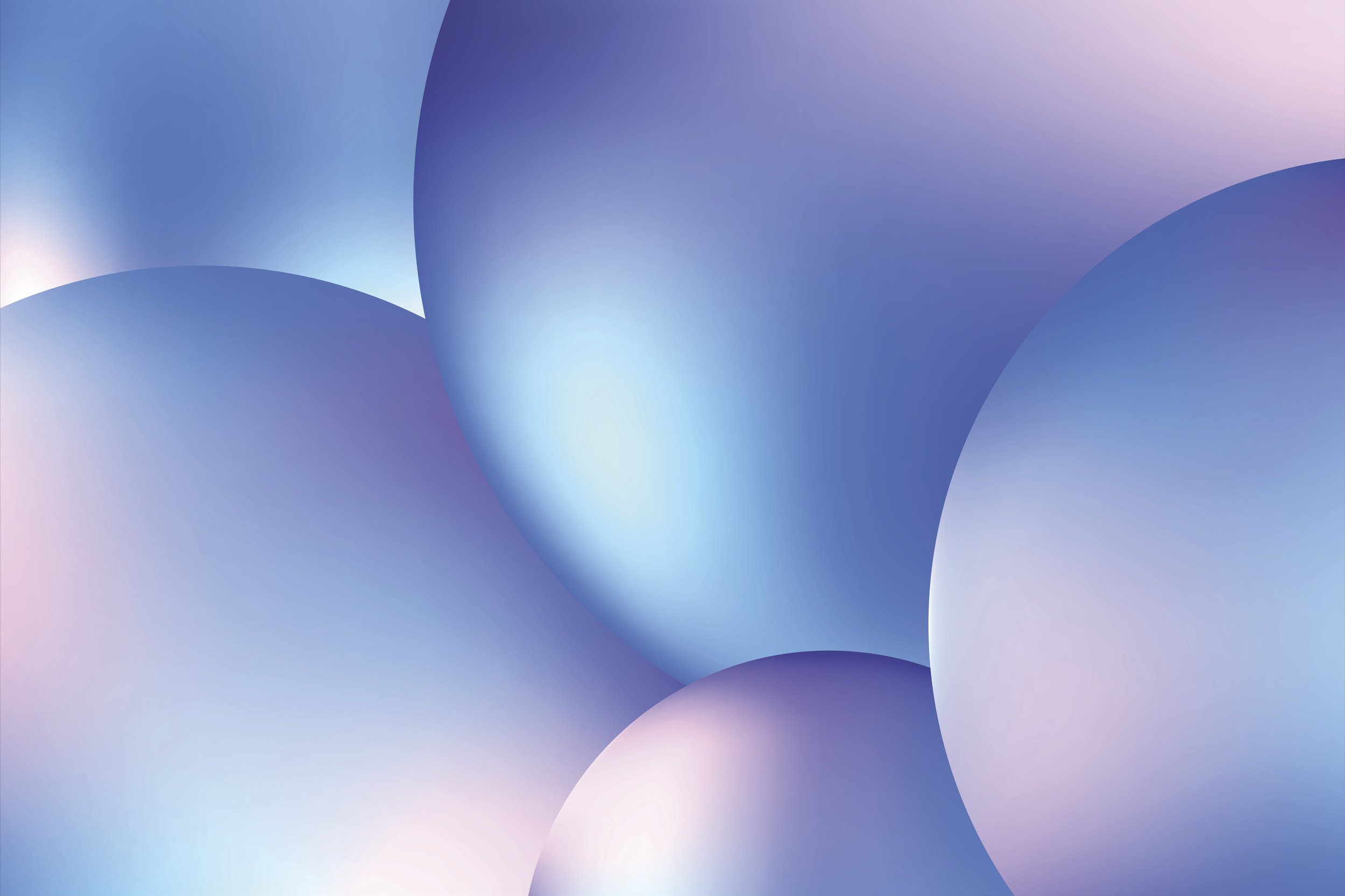
Modeling matter at extreme densities
at Texas A&M University
Jeremy W. Holt
Supported by
How investigating the properties of nuclear matter can lead to a better understanding of neutron stars and core-collapse supernovae
Highlighted works
-
Machine learning the nucleon - nucleon interaction (6/22/23)
How generative machine learning models are being used to model the nuclear force and associated uncertainties.
-
Neutrino interactions in hot and dense matter (6/8/23)
Understanding the role of nuclear collective excitations on neutrino propagation in core-collapse supernovae.
-
Neutron star bulk properties (4/19/22)
Combining observational, theoretical, and experimental investigations to understand the bulk properties of neutron stars.
-
Simulating heavy-ion collisions (7/28/21)
Studying charged pion production in relativistic heavy ion collisions from the Vlasov-Uehling-Uhlenbeck tranport model.
-
Machine learning for quantum many-body calculations (2/4/21)
How a new class of machine learning models (normalizing flows) is helping to better understand hot and dense nuclear matter.
-
Microscopic nuclear scattering and reactions (9/17/20)
Developing nucleon-nucleus scattering potentials from fundamental two-body and three-body forces.
Nuclear physics of high-energy astrophysical phenomena
Movie credit: NASA/Goddard Space Flight Center
Image credit: NASA/CXC/SAO